Pranav Agarwal
I’m a Machine-Learning Research Intern at Wayve, working on World Models for Autonomous driving.
I’m also finishing my Ph.D. at Mila (advised by Sheldon Andrews and Samira Ebrahimi Kahou), with a focus on reinforcement learning for robotics and character animation. My research explores efficient reinforcement learning algorithms using improved prior modeling for robotic applications. Specifically, I have worked on:
- World models (Transformer based) for sample-efficient reinforcement learning
- Automating reward modeling from large offline trajectories
- Continual Learning
- Applications in complex robotic systems, such as autonomous driving
- Interpreting decision-making of LLMs
Previously, I was a student researcher at Inria, collaborating with Natalia Díaz-Rodríguez and Raoul de Charette. I completed my Bachelor's in Electronics and Communication Engineering at IIIT Guwahati, where I was awarded the President's Gold Medal. During my undergraduate studies, I worked as a research intern at SUTD with Professor Gemma Roig.
Research Interests: Reinforcement Learning | Lifelong Learning | World Models | Video Models | Robotics | LLM Interpretability
Hobbies: Outside of research, I enjoy long walks, capturing nature (check out my photos), reading (see my book collection), and lifting weights.
I’m currently on the job market and open to full-time positions. I’m also happy to collaborate—feel free to reach out!
[
Email  / 
CV  / 
Github  / 
Twitter  / 
Google Scholar  / 
Linkedin / 
Projects
]
|
|
Experience & Education
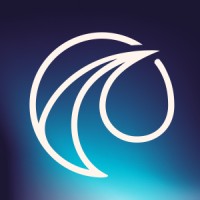 |
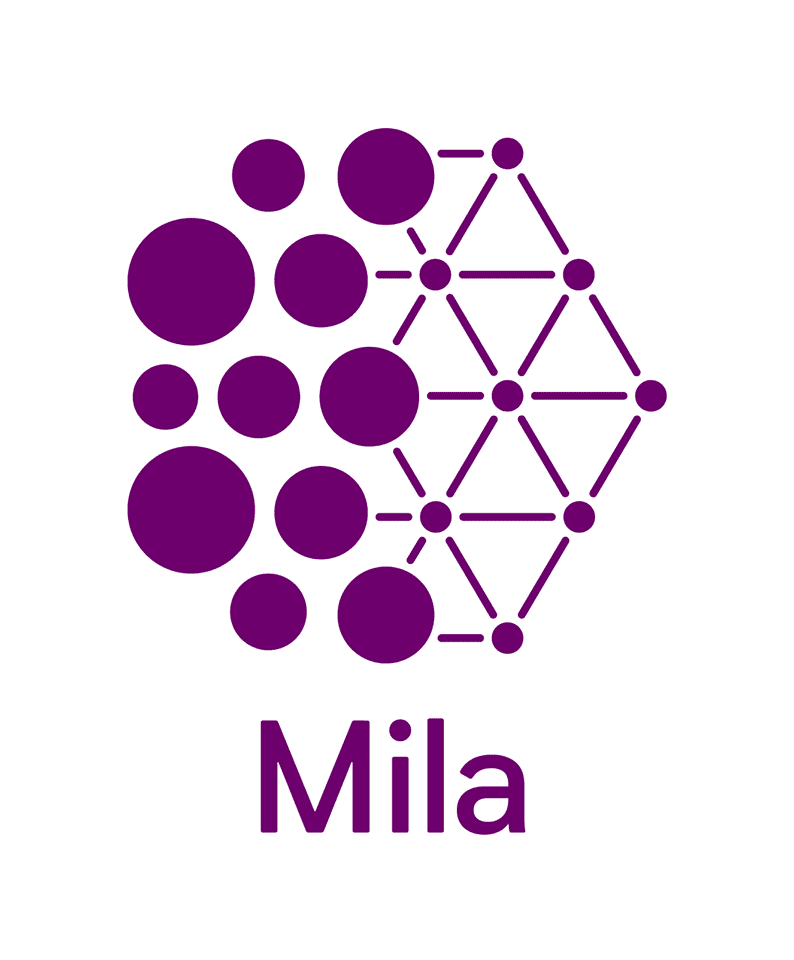 |
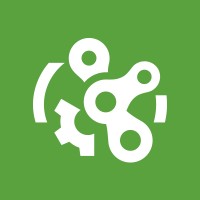 |
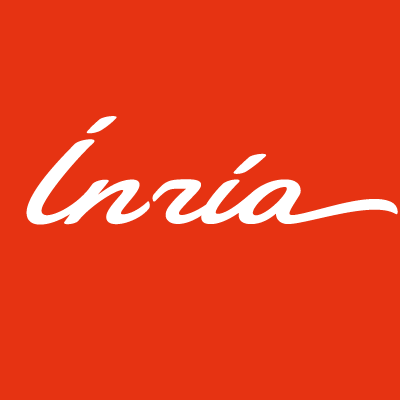 |
Machine-Learning Research Intern Wayve, Vancouver May 2025 – |
Graduate Researcher Mila, Québec Jan 2022 – |
Research Student CM-Labs Jan 2022 – Sept 2022 |
Research Assistant Inria, Paris May 2019 – Apr 2021 |
 |
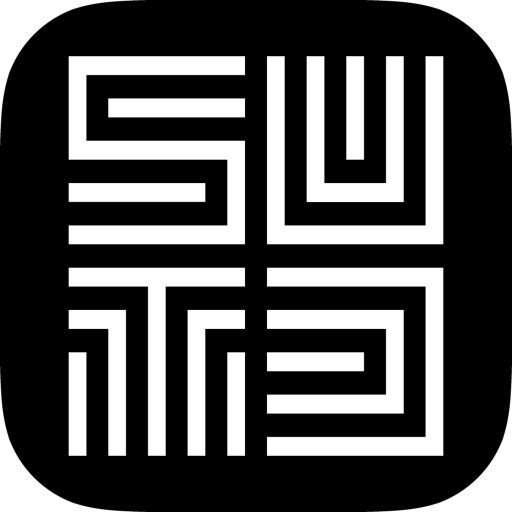 |
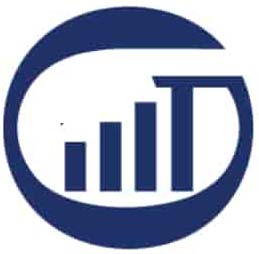 |
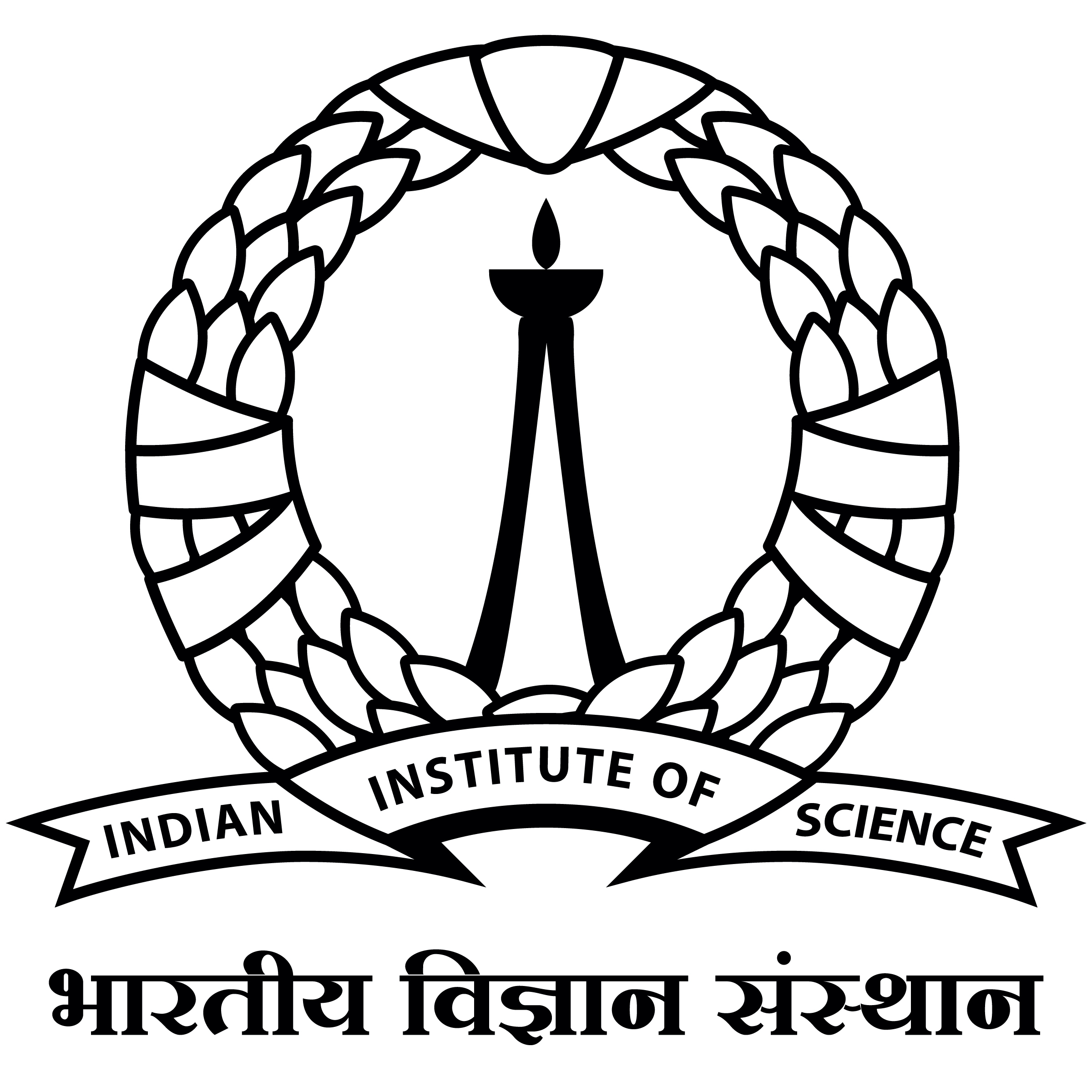 |
PhD Student ÉTS, Montréal Jan 2022 – |
Research Intern SUTD May 2018 – Aug 2018 |
B.Tech ECE IIIT Guwahati Aug 2015 – May 2019 |
Research Intern IISc, Bangalore May 2017 – Aug 2017 |
News
Research
|
Transformers in Reinforcement Learning: A Survey
Pranav Agarwal,
Aamer Abdul Rahman,
Pierre-Luc St-Charles,
Simon J.D. Prince,
Samira Ebrahimi Kahou
Under Review (2023)
Paper /
Webpage
This survey explores the impact of transformers in reinforcement learning, addressing common RL challenges, while examining their applications in representation learning, policy optimization, and interpretability.
|
|
Learning to Play Atari in a World of Tokens
Pranav Agarwal,
Sheldon Andrews,
Samira Ebrahimi Kahou
International Conference on Machine Learning (ICML), 2024.
Paper /
Code /
Webpage /
Slides
This work introduces Discrete Abstract Representations for Transformer-based Learning (DART), a sample-efficient method that utilizes discrete representations to improve world modeling and learning behavior in reinforcement learning, achieving superior performance on the Atari 100k benchmark compared to existing methods.
|
|
Empowering Clinicians with MeDT: A Framework for Sepsis Treatment
Aamer Abdul Rahman,
Pranav Agarwal,
Vincent Michalski,
Rita Noumeir,
Philippe Jouvet,
Samira Ebrahimi Kahou
NeurIPS 2023 Goal-Conditioned Reinforcement Learning Workshop (Spotlight).
Paper /
Code /
Webpage /
Slides
The Medical Decision Transformer (MeDT) leverages the transformer architecture to enhance offline reinforcement learning for sepsis treatment recommendations, utilizing a goal-conditioned RL paradigm that improves interpretability and clinician interactivity, while achieving competitive results on the MIMIC-III dataset.
|
|
TPTO: A Transformer-PPO based Task Offloading Solution for Edge Computing Environments
Niloofar Gholipour,
Marcos Dias de Assuncao,
Pranav Agarwal,
Julien Gascon-Samson,
Rajkumar Buyya,
IEEE 29th International Conference on Parallel and Distributed Systems (ICPADS).
Paper /
Code /
Webpage /
Slides
This paper introduces TPTO, a Deep Reinforcement Learning approach that utilizes Transformer and Proximal Policy Optimization to efficiently offload dependent IoT tasks to edge servers, significantly reducing latency for IoT applications compared to state-of-the-art methods.
|
|
Automatic Evaluation of Excavator Operators using Learned Reward Functions
Pranav Agarwal,
Marek Teichmann,
Sheldon Andrews,
Samira Ebrahimi Kahou
NeurIPS 2022 Reinforcement Learning for Real Life Workshop.
Paper /
Code /
Video /
Slides
A novel automatic evaluation strategy for excavator operators is proposed, utilizing machine dynamics and safety criteria, which is then validated through reinforcement learning in a simulation, resulting in safer and more realistic excavator maneuvering policies.
|
|
Goal-constrained Sparse Reinforcement Learning for End-to-End Driving
Pranav Agarwal,
Pierre de Beaucorps,
Raoul de Charette
In submission (2021).
Paper /
Code /
Video
A curriculum-based deep reinforcement learning approach for end-to-end driving is proposed, using sparse rewards and navigation view maps to achieve generalization on unseen roads and longer distances.
|
|
Egoshots, an ego-vision life-logging dataset and semantic fidelity metric to evaluate diversity in image captioning models
Pranav Agarwal,
Alejandro Betancourt,
Vana Panagiotou,
Natalia Diaz-Rodriguez
Machine Learning in Real Life (ML-IRL) ICLR 2020 Workshop.
Paper /
Code /
Video /
Slides
A new image captioning dataset, Egoshots, is introduced alongside a novel evaluation metric, Semantic Fidelity, to address biases in existing models and enable caption assessment without annotations.
|
|
Learning to synthesize faces using voice clips for Cross-Modal biometric matching
Pranav Agarwal,
Soumyajit Poddar,
Anakhi Hazarika,
Hafizur Rahaman
2019 IEEE Region 10 Symposium (TENSYMP).
Paper /
Code
A framework for cross-modal biometric matching is proposed, generating faces from voice clips using various generative networks, with RC-GAN achieving the best identity accuracy of 84.52% and VAE producing the highest quality images.
|
|